Implementing Effective Data Governance in Analytics Tracking
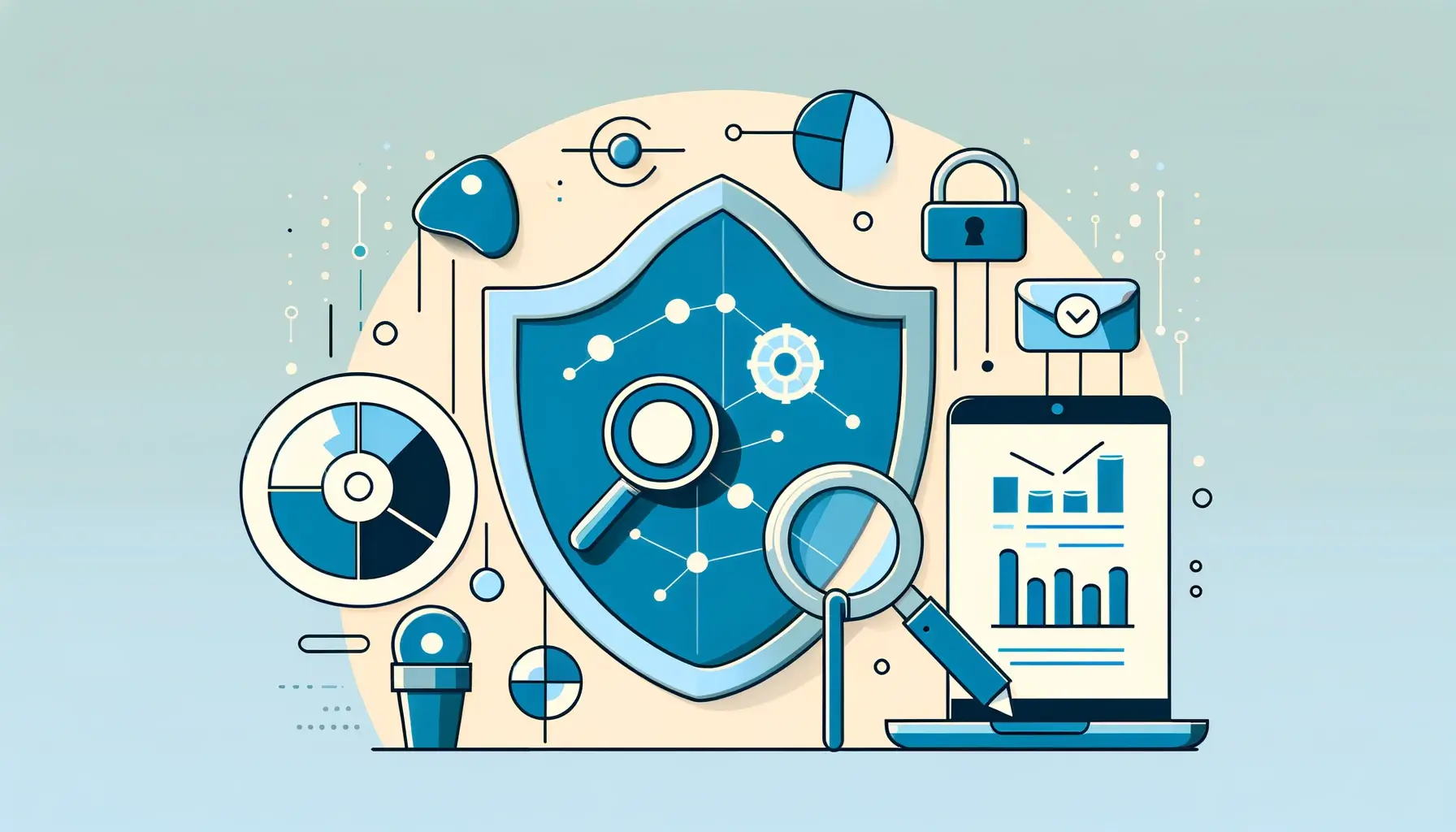
Table of Contents
ToggleData governance is the practice of managing data properly – it involves setting up rules and procedures to ensure that the data a company collects, uses, and stores is of high quality, secure, and used appropriately.
This article will explore the importance of data governance in analytics, detailing why it’s essential for making informed business decisions. We’ll also introduce some strategies and tools that can help organizations manage their data more effectively.
What is Data Governance in Analytics?
Think of data governance in analytics like the rules and plans a company follows to handle its data—the information it collects, uses, and stores. It’s all about making sure this data is good quality, safe, and used in the right way. This includes deciding who is responsible for what data, how to keep the data clean and accurate, and making sure the company follows laws about data privacy and security.
In analytics, when companies look at data to make decisions, having strong data governance means they can trust their data more. This trust helps them make better choices because they’re using reliable information.
Importance and Objectives of Data Governance
The importance of data governance cannot be overstated, especially in today’s data-driven world where organizations rely heavily on data analytics for strategic decision-making, operational efficiency, and competitive advantage.
Data governance ensures that data across the organization is accurate, accessible, consistent, and protected, which in turn supports:
- Compliance and Privacy: Following rules and privacy laws such as GDPR, CCPA, and others lowers the risk of data breaches and legal penalties.
- Data Quality: Ensures the data is correct and reliable, which is crucial for making smart decisions.
- Operational Efficiency: Streamlining data management processes to improve productivity and reduce costs associated with managing disparate data sources and correcting errors.
- Risk Management: Identifies and reduces risks related to handling data, like security threats or breaking rules.
- Strategic Decision-Making: With good data governance, businesses can trust their data for making big decisions, and spotting new chances for growth.
The primary objectives of data governance include:
- Establishing Clear Policies and Procedures: To manage data effectively across its lifecycle, from collection to deletion.
- Defining Roles and Responsibilities: To ensure accountability and clarity in data management processes.
- Enhancing Data Quality: To improve the accuracy, consistency, and trustworthiness of data.
- Ensuring Compliance: To meet legal and regulatory requirements related to data privacy and protection.
- Facilitating Data Sharing and Accessibility: To make data available to authorized users in an efficient and secure manner, thereby fostering innovation and collaboration.
Foundations of Data Governance and Analytics Tracking
When we dive into the foundations of data governance and analytics tracking, it’s like setting the stage for managing a vast and valuable collection of information. It involves understanding the core principles, deploying the right tools for monitoring, and ensuring the data is handled with care and precision.
Key Concepts in Data Governance
Understanding the treasure map of data governance is crucial. It’s all about the strategies and structures in place to ensure that data is trustworthy, understandable, and useful.
Data governance refers to the process of defining the responsibilities of individuals who oversee data, as well as the storage and access permissions.
Furthermore, it establishes policies and guidelines to ensure that data handling is conducted with integrity and respect, similar to a pirate’s code designed to protect data use and integrity.
Data governance is the lighthouse guiding the vast ships of the enterprise through the fog of digital transformation, ensuring safe passage by illuminating the rocks of risk and guiding us to the shores of strategic success.
Essentials of Data Governance in Analytics:
Analytics tracking utilizes advanced tools to monitor user interactions within a website or application. It systematically records user pathways, actions, and engagement levels, providing a comprehensive overview of the user journey.
This data is crucial for informed decision-making, enabling enhancements to the user experience and ensuring that digital platforms are both engaging and effective.
When analytics tracking utilizes tools such as Google Tag Manager (GTM) and Google Analytics (GA), establishing a structured and secure approach to data collection is imperative. Here’s a professional overview tailored to these considerations:
- Structured Naming Conventions: Adopting a standardized naming system for GTM/GA is crucial for maintaining organized and easily interpretable data and tags. This practice ensures a cohesive data environment, facilitating streamlined analysis and application.
- Careful Handling of Personally Identifiable Information (PII): Google outlines specific guidelines regarding the collection of PII, which encompasses any information capable of directly identifying an individual, such as:
- email addresses
- mailing addresses
- phone numbers
- precise locations (such as GPS coordinates – but see the note below)
- full names or usernames
Tips to Avoid Sharing Personal Info:
- In the UA Google Analytics, you could Create custom alerts.
- In the new Google Analytics 4, there isn’t a specific tool for this yet, but you can be careful about what gets sent from GTM.
- In GTM, there’s a special setup called “Redactor PII variable” that helps hide personal info.
Developing a Data Governance Framework
Creating a data governance framework is like building a solid foundation for how a company handles its data. It’s all about making a plan, setting up a team, and putting the right rules in place. Let’s break it down:
Strategy, Structure, and Roles
This step is about planning your data management:
- Making a Plan: Decide what you want to achieve with your data. This is about setting goals for how data should be used and managed.
- Organizing the Team: Create a clear structure that defines who is responsible for what. This ensures everyone knows their role in managing data.
- Assigning Responsibilities: Give specific tasks to team members. This includes deciding who will oversee data management, who will handle data security, and who will use the data.
Data Quality and Privacy Management
This focuses on keeping data accurate and secure:
- Keeping Data Clean: Make sure your data is correct and up-to-date. This means checking data regularly and fixing any errors.
- Protecting Privacy: Keep personal data safe. This involves making sure only authorized people can access and use personal data.
Security Measures and Compliance with Regulations
This involves protecting data and following legal rules:
- Setting Up Security: Use technology and methods to keep data safe from unauthorized access or data breaches.
- Following Legal Rules: Make sure your data practices comply with laws to avoid fines and ensure you’re using data correctly.
Implementing Data Governance
Operationalizing data governance specifically within the realm of analytics tracking involves translating strategic data governance principles into actionable practices that enhance the accuracy, security, and ethical use of analytics data. Here’s a focused approach to how this can be achieved:
Technologies and Tools for Data Management
Technological solutions play a significant role in operationalizing data governance.
Employing advanced technologies and tools is crucial for efficient data management within analytics tracking. These include:
Data Integration Tools: Essential for aggregating data from various analytics tracking sources into a centralized platform for comprehensive analysis. (Example: Informatica)
Data Quality Tools: Deploy tools specifically designed to assess and improve the quality of data derived from analytics tracking, ensuring it is accurate and reliable for decision-making. (Example: Talend)
Data Security Tools: Use security tools to protect analytics data from unauthorized access, ensuring that data collected through tracking is securely stored and transmitted. (Example: Symantec Data Loss Prevention)
Compliance Management Tools: Implement tools to monitor and ensure compliance with relevant data protection regulations in analytics practices, aiding in the management of consent and privacy settings. (Example: IBM OpenPages)
Conclusion
Creating a solid data governance plan is critical for any company that wants to get the most out of its data. We’ve viewed that it’s all about having clear standards, ensuring that the data is good and safe, and utilizing the appropriate technologies.
As things progress, staying current with data governance will help businesses stay on top of their game. It ensures that their data is always something they can rely on to make significant decisions and move forward.